Data Science
Build a model that helps detect Credit Card Fraud using logistic regression
Focus is on building a model to detect credit card fraud using logistic regression, a crucial tool in the fight against digital financial fraud.
Certified by
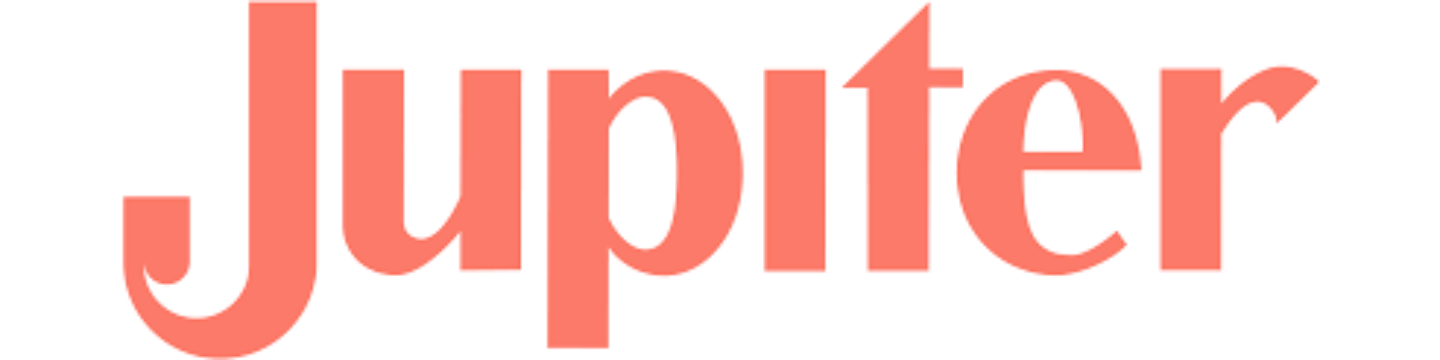
Role
Data Scientist
Industry
Finance
No. of Subscribers
5
Level
Intermediate
Time Commitment
60 Hours
Duration
30 days
Tools you’ll learn
Here’s What You Work On
About the Company
Jupiter is a digital banking application that provides convenient, effective customer service with intelligent features that ensure the user’s financial health is always A plus.
Their vision is to Empower people to achieve financial wellness.
In this experience, we focus on building a model to detect credit card fraud using logistic regression, a crucial tool in the fight against digital financial fraud.
Their vision is to Empower people to achieve financial wellness.
In this experience, we focus on building a model to detect credit card fraud using logistic regression, a crucial tool in the fight against digital financial fraud.
Explore
the following work techniques
Logistic Regression
Python Libraries
Bridging the gap
This project involves building a logistic regression model to detect fraudulent credit card transactions.
The dataset contains information about credit card transactions, including the transaction amount, time, and several anonymized features, along with a binary target variable indicating whether the transaction is fraudulent or not.
The experience is divided into four main components: data preprocessing and cleaning, feature selection and engineering, model training and evaluation, and interpretation and communication of results.
The dataset contains information about credit card transactions, including the transaction amount, time, and several anonymized features, along with a binary target variable indicating whether the transaction is fraudulent or not.
The experience is divided into four main components: data preprocessing and cleaning, feature selection and engineering, model training and evaluation, and interpretation and communication of results.
Apply
the following skills
Data Analysis & Modelling
Data preparation
Expected output
As an outcome to this experience, you are required to submit your model, along with a presentation that showcases your analysis and model application.
Create
the following deliverables
Well documented Jupyter Notebook
Presentation on key findings and model evaluation
What you’ll need before starting
Python Libraries.
Experience with Logistic Regression
Experience with Logistic Regression